by Mathieu Vrac
Global climate models (GCMs), which simulate the Earth’s climate system in various ways, are crucial for understanding and anticipating climate change. Typically, these models are combined into multi-model ensembles (MMEs) to distill a robust signal from diverse simulations and outputs. These MMEs are summarized using methods such as multi-model means, medians, or quantiles, sometimes with weighted adjustments. A traditional alternative to improve the realism of the climate projections consists in bias-correcting the different simulations. These combination or correction approaches are usually exclusive and thus concern separate communities.
An international group of researchers from LSCE, INRAE and Univ. of Lausanne has developed a new method to enhance the quality of the climate change projections, based on an original concept hybriding the notions of model combination and bias correction.
Their study introduces an innovative probability aggregation method called “alpha pooling”, building an aggregated cumulative distribution function (CDF) that closely aligns with a reference CDF during the historical calibration period. The aggregated CDFs can then be used for bias adjustment of raw climate simulations, thus achieving the first “multi-model bias correction”. In practice, each CDF undergoes a non-linear transformation based on a parameter α. Each transformed CDF is assigned a weight, which increases with its closeness to the reference transformed CDF. The key to alpha pooling is the parameter α, which dictates the transformation type and, consequently, the aggregation type, hence generalizing both linear and log-linear pooling methods.
The research confirms that alpha pooling is a valid aggregation method by verifying certain optimal properties. Focusing on climate model simulations of temperature and precipitation over Western Europe, the study conducts several experiments to compare the performance of alpha pooling against current methods, including multi-model means and weighted variants. These experiments include a reanalysis-based evaluation, a perfect model experiment (see figure), and a sensitivity analysis of the climate models. The results demonstrate the superiority of the alpha pooling method, highlighting its effectiveness in combining GCM CDFs. The study’s findings suggest that this novel CDF pooling strategy for multi-model bias correction is a viable alternative to traditional GCM-by-GCM bias correction methods, as it efficiently handles and integrates multiple climate models simultaneously.
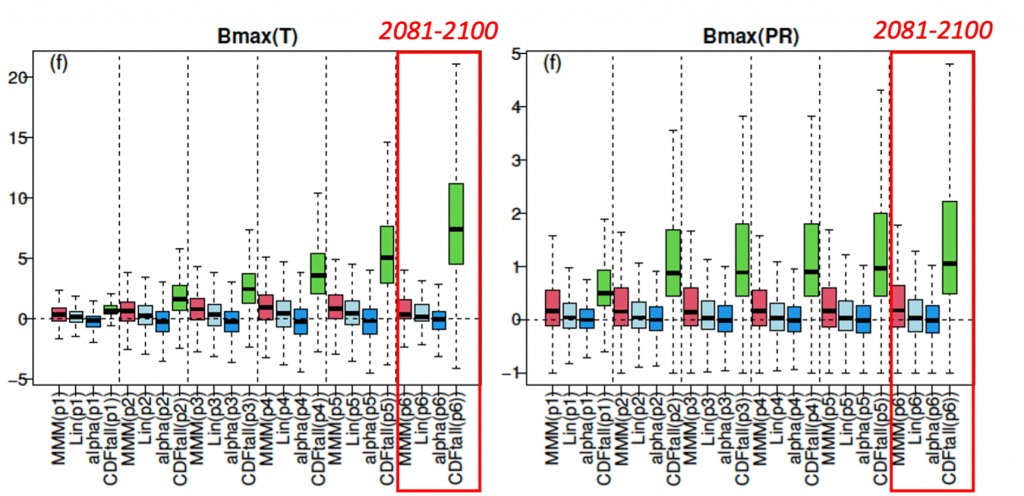
Reference: Vrac, M., Allard, D., Mariéthoz, G., Thao, S., Schmutz, L. (2024) Distribution-based pooling for combination and multi-model bias correction of climate simulations. Earth System Dynamics, 15, 735–762, https://doi.org/10.5194/esd-15-735-2024.
Contact: