Presentation
The understanding and modeling of climate and environmental variability at various spatial scales, covering a spectrum from large atmospheric structures to local phenomena, is one of the missions of the LSCE. It also extends over different temporal scales, encompassing the study of past climates, current processes, and future developments. At the heart of this innovative endeavor, the ESTIMR team uses and develops cutting-edge artificial intelligence methods, specifically tailored to climate challenges. This work is based on close multidisciplinary collaboration, involving experts in climatology, modeling, physics, and statistics.
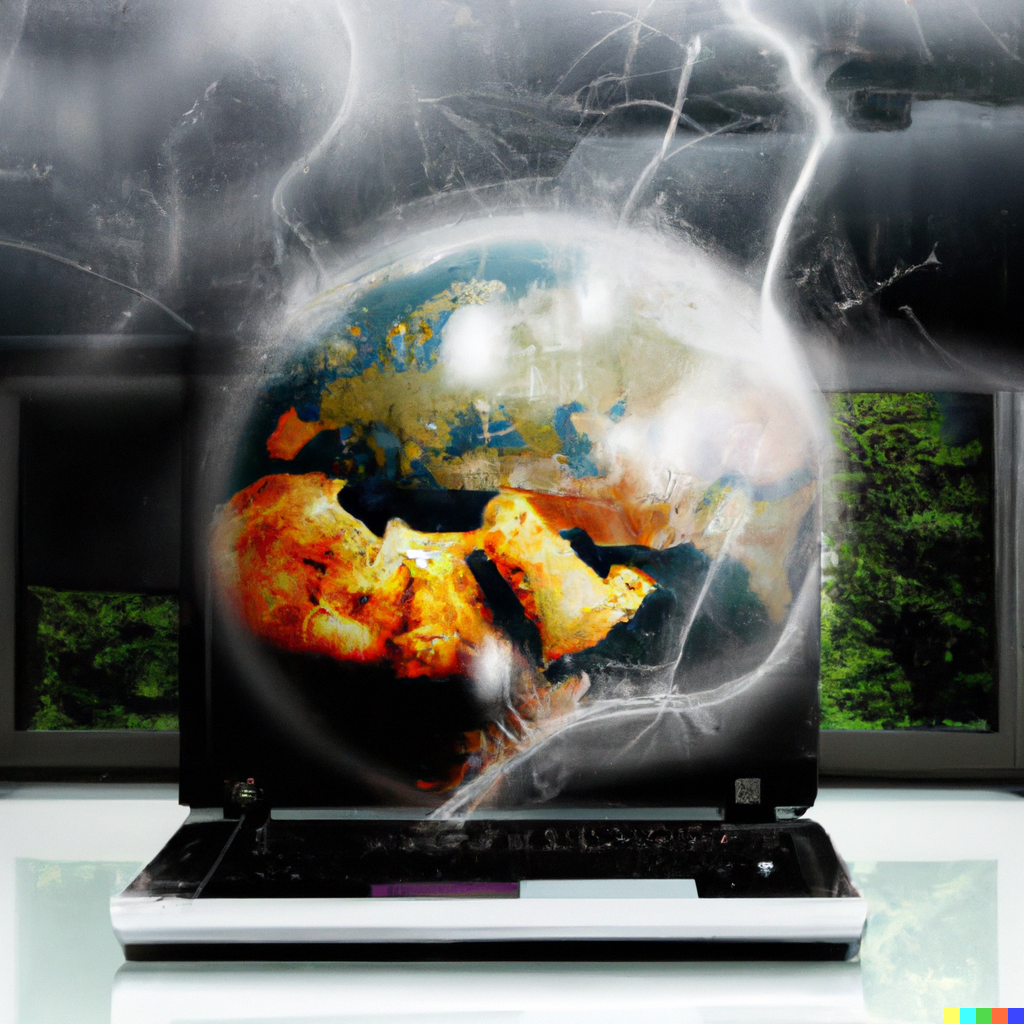
The integration of artificial intelligence also extends to the field of data assimilation in geosciences. The work of Lafon et al. (2023) focuses on the uncertainty associated with learning dynamic models and solvers using variational methods. Their proposal consists of an end-to-end neural scheme based on a variational Bayesian inference formulation, aiming to simultaneously address data assimilation and uncertainty quantification. This work demonstrates the relevance of their approach through experiments conducted on dynamics such as the Lorenz model (1963) and river discharge measurements.
From a different perspective, François et al. (2021) research focuses on adjusting the spatial dependence of climate model outputs. They use cycle-consistent adversarial networks to correct the spatial properties of climate simulations. Their algorithm, MBC-CycleGAN, employs a computer vision technique to perform this correction. Results indicate that MBC-CycleGAN produces reasonable adjustments of spatial correlations for temperature and precipitation, compared to other bias correction methods.
Lastly, Fery et al. (2022) explore the learning of a meteorological dictionary of atmospheric patterns using Latent Dirichlet Allocation (LDA). This innovative approach utilizes LDA, initially employed in linguistics for topic modeling, to construct a meteorological dictionary. Documents are defined as daily maps of a target observable, with words being the grid points composing the map. LDA provides a representation of documents in terms of combinations of spatial patterns called motifs, latent patterns inferred from the ensemble of snapshots. For atmospheric data, motifs correspond to pure synoptic objects such as cyclones and anticyclones, considered as constitutive elements of weather regimes. Results demonstrate that LDA weights provide a natural way to characterize the impact of climate change on the recurrence of patterns associated with extreme events.
In conclusion, artificial intelligence emerges as a central and indispensable player at LSCE for the comprehensive understanding of complex climate phenomena. This innovative approach, leveraging advanced statistical modeling, data assimilation, and machine learning methods, represents an essential pillar in efforts to grasp the complexity of climate dynamics. The judicious use of artificial intelligence at LSCE enables the exploration of climate models with unparalleled precision and resolution, thus facilitating the capture and analysis of atmospheric variations at different spatial and temporal scales. These technological advancements are crucial in proactive climate risk management, providing sophisticated tools to anticipate, mitigate, and adapt to environmental changes. The significant contribution of artificial intelligence also manifests in its ability to handle massive and complex data sets, allowing for more precise assimilation of climate information. This data assimilation greatly enhances model quality, thereby improving the reliability of climate projections. These advances play a crucial role in elucidating the implications of climate change on both local and global scales, thus providing a solid foundation for informed environmental policy decisions. In summary, artificial intelligence at LSCE not only plays a central role in understanding complex climate phenomena but also opens up new perspectives to address urgent challenges related to climate change.
- Lafon, N., Fablet, R., & Naveau, P. (2023). Uncertainty Quantification When Learning Dynamical Models and Solvers With Variational Methods. *Journal of Advances in Modeling Earth Systems, 15*(11), e2022MS003446. [DOI: 10.1029/2022MS003446](https://doi.org/10.1029/2022MS003446)
- François, B., Thao, S., & Vrac, M. (2021). Adjusting spatial dependence of climate model outputs with cycle-consistent adversarial networks. Climate Dynamics, 57, 3323-3353. [DOI: 10.1007/s00382-021-05869-8](https://doi.org/10.1007/s00382-021-05869-8)
- Fery, L., Dubrulle, B., Podvin, B., Pons, F., & Faranda, D. (2022). Learning a weather dictionary of atmospheric patterns using Latent Dirichlet Allocation. *Geophysical Research Letters, 49*. [DOI: 10.1029/2021GL096184](https://doi.org/10.1029/2021GL096184)