Nos thématiques
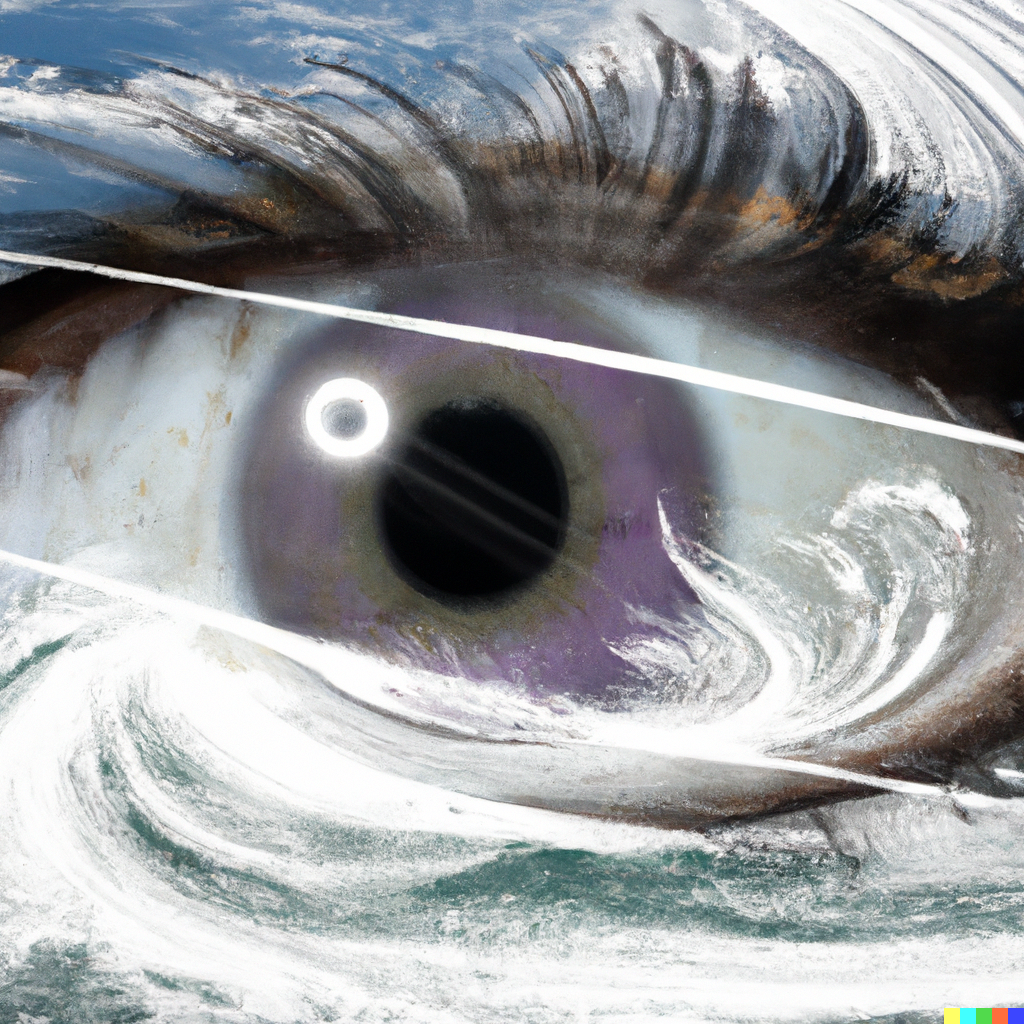
Nos outils
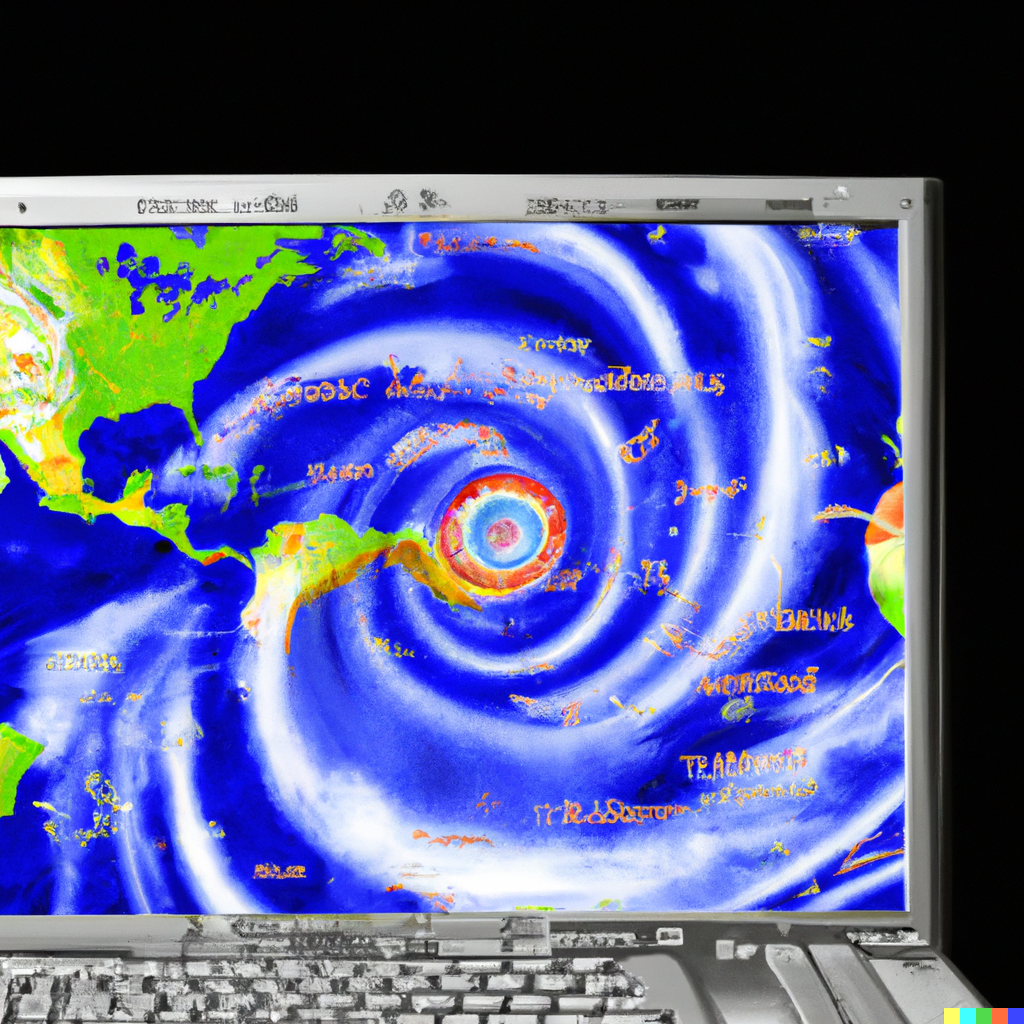
Notre équipe
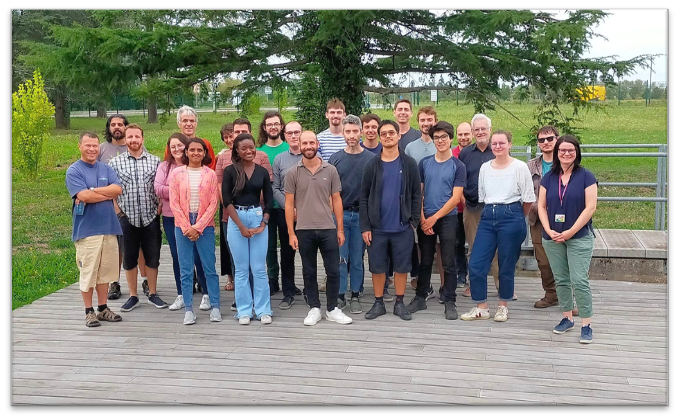
Nous rejoindre
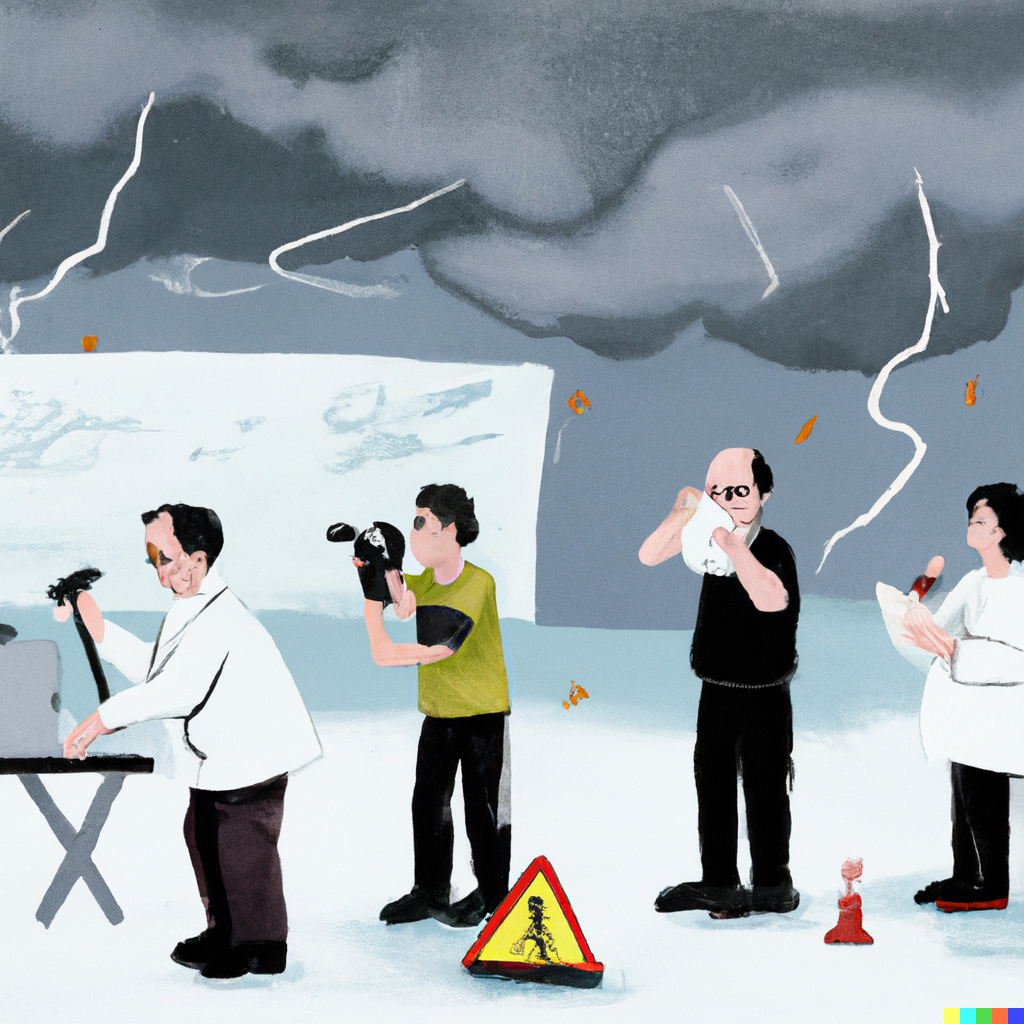
À la Une
-
L’empreinte du changement climatique sur les inondations au Portugal et les tempêtes de poussière saharienne en mars 2024
Une étude récente révèle que le changement climatique a amplifié un événement météorologique extrême ayant touché l’Europe fin mars 2024. Meteosat-8 HRV, 28 July 2005, 07:00 UTC Une étude récemment publiée dans Journal of Geophysical Research: Atmospheres par une équipe internationale impliquant le Laboratoire des Sciences du Climat et de l’Environnement (LSCE), l’Institut National de…