by M. Vrac, S. Thao, P. Yiou
Should Multivariate Bias Corrections of Climate Simulations Account for Changes of Rank Correlation Over Time?
by M. Vrac, S. Thao, P. Yiou
Inter-variable dependencies are key properties to characterize many climate phenomena such – as compound events—and their future changes. Yet, climate simulations often have statistical biases. Hence, univariate (1dBC) and multivariate bias correction (MBC) methods are regularly applied but Inter-variable properties (e.g., correlations) can be altered by BC corrections. Then, it is necessary to assess how underlying assumptions of BC methods on climate change affect the adjustments. This can lead to better choices of BC methods.
Here, we investigated whether an MBC method should try reproducing, preserving or modifying the changes in rank correlations between daily temperature and precipitation over Europe. To do so, we set up an original “perfect model experiment” that we applied to two different climate simulation ensembles over 2001–2100: 40 runs from the Community Earth System Model (CESM) global climate model and 11 runs from the Coupled Models Intercomparison Project (CMIP6) exercise.
Within this experiment, we consider one model as a possible truth (i.e., reference) to evaluate the Stationarity (St), non-stationarity (NSt), no-correction (Raw) or multi-model mean climate change (CC) assumptions to perform bias correction of the intervariable correlation. Hence, our approach consists in taking one model (or run) as reference and evaluate the four assumptions on the other models (resp. runs) with respect to the reference one. The same procedure is repeated for another reference model until all models (resp. runs) have each served once as reference.
The results highlight that, within the multi-run single Global Climate Models ensemble (CESM), accounting for correlation changes brings valuable information for long-term projections but that a stationarity assumption provides less biased correlations, up to medium-term projections (2060). For the multi-model ensemble (CMIP6), the non-stationarity assumption provides larger biases than the stationarity approach, up to the end of the century. Not correcting the model rank correlations (1dBC) provides the worst results. Whenever an ensemble is available, the best results come from accounting for the “robust” part of the change signal (i.e., average change from different runs).
These findings plead for using ensembles and their robust information, in order to perform robust (multivariate) bias corrections.
Reference
Vrac, M., Thao, S., & Yiou, P. (2022). Should multivariate bias corrections of climate simulations account for changes of rank correlation over time? Journal of Geophysical Research: Atmospheres, 127, e2022JD036562. https://doi.org/10.1029/2022JD036562
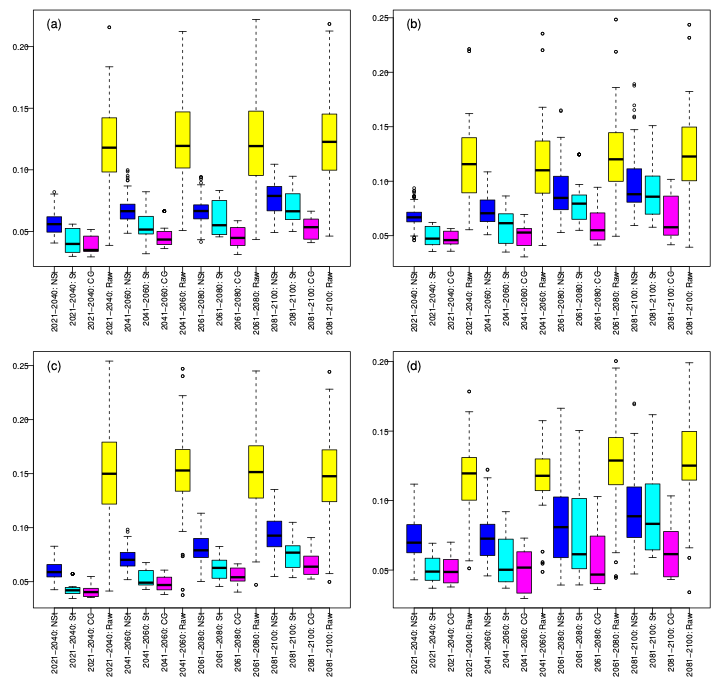